Data Analytics for Beginners
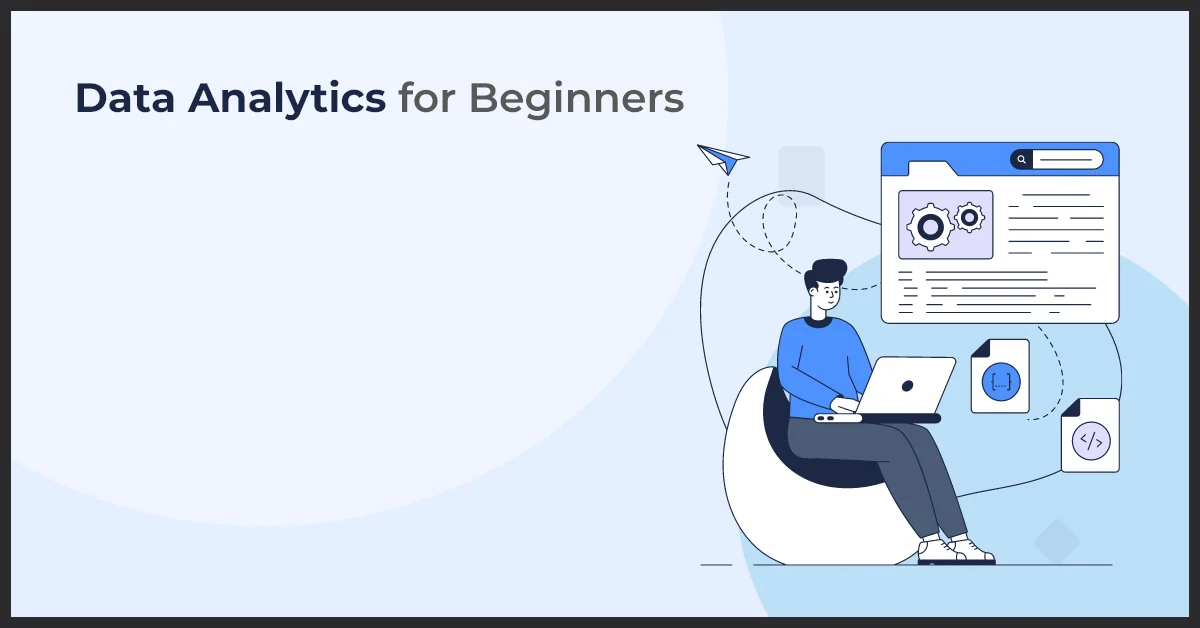
Published on: November 1, 2023
Updated on: June 25, 2024
1171 Views
- Analytics
27 min read
Welcome to our comprehensive guide on data analytics for beginners. In today's digital age, the ability to gather, analyze, and interpret data has become crucial for organizations across various industries. By harnessing the power of data analytics, businesses can gain valuable insights and make informed decisions that drive growth and success.
Data analytics refers to the process of examining raw data to uncover patterns, draw conclusions, and make predictions. It involves collecting and organizing data, applying statistical techniques and algorithms, and using visualization tools to present findings in a meaningful way.
The importance of data analytics cannot be emphasized enough. It allows companies to understand customer behavior, identify trends, detect opportunities, and mitigate risks. With data analytics, organizations can optimize their processes, improve operational efficiency, and gain a competitive advantage in the market.
One of the key benefits of data analytics is its ability to aid decision-making. By analyzing large volumes of data, businesses can make data-driven decisions rather than relying on guesswork or intuition. Data analytics helps identify the most effective marketing strategies, optimize pricing models, and improve product offerings based on customer preferences.
Data analytics plays a pivotal role in various industries, including finance, healthcare, e-commerce, manufacturing, and more. In finance, it helps in fraud detection, risk assessment, and portfolio management. In healthcare, data analytics aids in patient diagnosis, resource allocation, and personalized medicine. In e-commerce, it enables personalized recommendations, targeted marketing, and supply chain optimization. The applications of data analytics are endless, and its potential for transforming industries is immense.
Whether you're a budding entrepreneur, a student exploring career options, or simply curious about the world of data analytics, this beginner's guide will provide you with the foundation you need. Get ready to unlock the power of data analytics and embark on a journey of insights and innovation.
Basic Concepts and Terminology in Data Analytics
As a beginner in the field of data analytics, it is essential to understand some basic concepts and terminology. These concepts will provide a solid foundation for your journey in the world of data analysis.
Data, Information, and Knowledge
In data analytics, data, information, and knowledge are interconnected but distinct concepts. Data refers to raw facts or figures that have no context or meaning on their own. Information is the result of processing and organizing data into a meaningful form. Knowledge, on the other hand, is the understanding and insight gained from analyzing information.
Types of Data: Structured vs. Unstructured
Data can be categorized into two types: structured and unstructured. Structured data is organized, formatted, and easily searchable. It is typically found in databases and spreadsheets. Unstructured data, on the other hand, is not organized in a predefined manner, making it challenging to analyze. Examples of unstructured data include social media posts, emails, and videos.
Descriptive, Predictive, and Prescriptive Analytics
Data analytics involves different types of analytics, including descriptive, predictive, and prescriptive analytics. Descriptive analytics focuses on understanding past data and providing insights into what has happened. Predictive analytics uses historical data to make predictions about future events or trends. Prescriptive analytics goes a step further and provides recommendations or actions to optimize outcomes based on predictive models.
Key Terminology: Algorithms, Models, and Metrics
Algorithms are step-by-step instructions or procedures used to perform specific tasks, such as data analysis. They are the backbone of data analytics. Models, on the other hand, are representations of the real-world system or process being analyzed. They help data analysts understand and make predictions or decisions based on data. Metrics are the measurements used to evaluate the performance or success of a data analytics project. They provide quantitative insights into the effectiveness of analytics techniques.
By grasping these basic concepts and terminology, you are now equipped to dive deeper into the world of data analytics. The next section will explore the importance and benefits of data analytics.
Importance and Benefits of Data Analytics
In today's data-driven world, data analytics plays a crucial role in various industries and organizations. By harnessing the power of data, businesses can gain valuable insights that can lead to improved decision making, greater efficiency, and a competitive advantage.
Improved decision making with data-driven insights
Data analytics allows companies to make informed decisions based on evidence and facts rather than relying on gut feelings or intuition. By analyzing large volumes of data, businesses can identify patterns, trends, and correlations that can help them make strategic decisions that drive growth and success.
Identifying patterns and trends
Data analytics enables businesses to identify patterns and trends within their data, which can uncover hidden insights and opportunities. By understanding customer behavior, preferences, and market trends, companies can optimize their strategies and tailor their products or services to meet the needs of their target audience.
Optimizing processes and resource allocation
Data analytics helps businesses optimize their processes and allocate resources more efficiently. By analyzing data, companies can identify bottlenecks, inefficiencies, and areas of improvement. This enables them to streamline operations, reduce costs, and improve overall productivity.
Gaining a competitive advantage
Data analytics provides businesses with a competitive advantage by uncovering insights that competitors may overlook. By leveraging data analytics, companies can stay ahead of the curve, identify emerging trends, and make proactive decisions that give them a competitive edge in the market.
Enhancing customer understanding and personalization
Data analytics allows businesses to gain a deeper understanding of their customers. By analyzing customer data, companies can segment their audience, personalize their marketing campaigns, and deliver targeted experiences that resonate with their customers on a personal level. This leads to greater customer satisfaction, loyalty, and ultimately, increased revenue.
- Improved decision making with data-driven insights
- Identifying patterns and trends
- Optimizing processes and resource allocation
- Gaining a competitive advantage
- Enhancing customer understanding and personalization
Data Collection and Data Types
Sources of Data: Internal vs. External
Data for analysis can come from different sources – internal and external. Internal data refers to data generated within an organization, such as sales records, customer databases, or employee performance metrics. External data, on the other hand, comes from outside the organization, such as market research reports, government data, or social media data.
Primary vs. Secondary Data
Primary data is collected directly from original sources through surveys, observations, or experiments. It is specific to the research question or objective and is gathered firsthand. Secondary data, on the other hand, is data that has been collected by someone else for another purpose but can be used for analysis. It includes data from publications, databases, or reports.
Quantitative and Qualitative Data
Data can be classified into two main types: quantitative and qualitative. Quantitative data is numerical and measurable, such as sales figures or website traffic. It focuses on the "what" and "how much" aspects of data. On the other hand, qualitative data is descriptive and subjective, providing insights into behaviors, opinions, or experiences. It focuses on the "why" and "how" aspects of data.
Data Sampling and Sampling Techniques
Data sampling is the process of selecting a subset of data from a larger population to analyze. It is often necessary due to the volume and complexity of data. Various sampling techniques, such as random sampling, stratified sampling, or cluster sampling, are used to ensure the sample represents the population accurately and avoids bias.
Data Cleaning and Preprocessing Techniques
Data cleaning is an essential step in the data analytics process. It involves removing or correcting errors, inconsistencies, and irrelevant information from a dataset. By cleaning the data, analysts ensure the accuracy and reliability of their analyses. Here are some important techniques for data cleaning and preprocessing:
Importance of Data Cleaning
Data cleaning is crucial because raw data often contains errors, duplicate records, and inconsistencies. These issues can lead to inaccurate analysis and misleading conclusions. By thoroughly cleaning the data, analysts can minimize the impact of these problems and improve the quality of their results.
Handling Missing Data
Missing data is a common challenge in data analytics. Analysts must decide how to handle missing values appropriately. They can either remove the records with missing data or impute values based on statistical methods such as mean, median, or regression techniques.
Dealing with Outliers and Noise
Outliers are extreme values that deviate significantly from the average or expected pattern in a dataset. Noise refers to irrelevant or random fluctuations in the data. Both outliers and noise can distort analysis results. Analysts can identify and handle outliers by using statistical techniques such as z-scores or boxplots. Noise can be reduced by applying smoothing techniques or filtering algorithms.
Data Transformation and Normalization
Data transformation involves converting data into a more suitable format for analysis. It includes techniques such as scaling, logarithmic transformation, and power transformation. Normalization, on the other hand, is the process of rescaling variables to a standard range. These techniques help improve the distribution and comparability of data.
Exploratory Data Analysis (EDA)
Exploratory Data Analysis (EDA) is an essential step in the data analytics process. It involves understanding the structure and nature of the data to gain insights and identify patterns. By analyzing the data visually and statistically, EDA helps uncover relationships and trends that can guide further analysis and decision-making.
Descriptive statistics and visualization are key techniques used in EDA. Descriptive statistics summarize the main characteristics of the data, such as measures of central tendency (mean, median) and measures of dispersion (standard deviation, range).
Visualization plays a crucial role in EDA as it helps represent data visually, making it easier to understand and interpret. Histograms are commonly used to visualize the distribution of a single variable, while box plots provide a visual summary of the distribution and identify outliers.
Scatter plots are used for bivariate analysis, allowing us to examine the relationship between two variables. They help identify patterns and potential correlations, such as positive or negative associations.
Exploratory data analysis is a powerful tool for beginners in data analytics. It enables a deeper understanding of the data, uncovers important insights, and supports informed decision-making. By utilizing techniques like histograms, box plots, and scatter plots, beginners can gain valuable insights from their data and pave the way for further analysis.
Statistical Analysis and Hypothesis Testing
In data analytics, statistical analysis is a crucial step in understanding and deriving meaningful insights from data. It involves the use of various statistical techniques to analyze, interpret, and draw conclusions from data.
One important concept in statistical analysis is hypothesis testing. Hypothesis testing is a statistical procedure used to test a hypothesis about a population parameter. There are two types of hypotheses: the null hypothesis (H0) and the alternative hypothesis (HA).
Types of Hypotheses: Null and Alternative
The null hypothesis is a statement that assumes there is no significant difference or relationship between variables. It represents the status quo or the default assumption.
The alternative hypothesis, on the other hand, is a statement that contradicts or challenges the null hypothesis. It suggests that there is a significant difference or relationship between variables.
Commonly used Statistical Tests
There are various statistical tests used in data analytics to determine the validity of the hypotheses. Some commonly used statistical tests include:
- T-Test: Used to compare the means of two groups or samples.
- Anova: Used to compare means of three or more groups.
- Chi-Square Test: Used to analyze categorical data and test for independence or association between variables.
- Regression Analysis: Used to determine the relationship between a dependent variable and one or more independent variables.
Interpreting the results of Hypothesis Testing
The interpretation of the results of hypothesis testing depends on the p-value obtained. The p-value is a measure of the strength of evidence against the null hypothesis. A small p-value (typically less than 0.05) indicates strong evidence against the null hypothesis, leading to its rejection in favor of the alternative hypothesis. On the other hand, a large p-value suggests weak evidence against the null hypothesis, leading to its acceptance.
It is important to note that hypothesis testing provides statistical evidence for or against a hypothesis, but it does not prove or disprove the hypothesis conclusively. It is always subject to the limitations of data collection and assumptions made.
Introduction to Data Visualization
Data visualization is a crucial aspect of data analytics that helps in effectively analyzing, understanding, and communicating data findings. Through visual representations, complex datasets can be simplified and presented in a way that is easy to grasp for both experts and beginners.
Importance of Data Visualization
Data visualization plays a vital role in data analytics as it allows beginners to interpret data quickly and make informed decisions. It enables the identification of patterns, trends, and correlations that may otherwise go unnoticed in raw data.
By presenting data visually, beginners can gain meaningful insights, discover relationships, and detect anomalies in the data. Visualizations also enhance the understanding of complex concepts, making it easier to communicate findings to stakeholders.
Types of Data Visualizations
Data visualizations come in various forms, each serving a different purpose. Some commonly used types of data visualizations include:
- Bar charts: Presents categorical data using rectangular bars to compare values.
- Line graphs: Illustrates trends and changes over time, typically using connected data points.
- Pie charts: Displays proportions of a whole using slices of a circle.
- Scatter plots: Shows the relationship between two variables through dots on a graph.
- Heatmaps: Represents data using colors on a grid, making it easier to identify patterns.
- And many more!
Choosing the Right Visualization
When selecting a data visualization technique, beginners must consider the objective and the type of data they want to present. Different visualizations are suitable for different scenarios, such as comparing values, showing trends, or presenting proportions.
It is essential to choose a visualization that effectively represents the data while being easy to understand. By selecting the right visualization, beginners can convey their findings accurately and efficiently.
Tools and Software for Data Visualization
There are several tools and software available that can assist beginners in creating stunning data visualizations. Some popular options include:
- Tableau: A powerful data visualization tool with a user-friendly interface.
- Power BI: Microsoft's business analytics tool that enables interactive visualizations and reports.
- Google Data Studio: A free tool for creating customizable dashboards and reports.
- RapidMiner: An open-source data science platform with built-in visualization capabilities.
These tools provide users with a range of features and functionalities to create compelling visualizations, even for those with limited coding skills.
Basics of Predictive Modeling and Machine Learning
Predictive modeling and machine learning are two key concepts in the field of data analytics. These techniques enable businesses and individuals to make accurate predictions and gain valuable insights from their data. In this section, we will introduce you to the basics of predictive modeling and machine learning.
Introduction to Predictive Modeling
Predictive modeling is a technique used to make predictions or forecasts based on historical data. It involves the use of mathematical and statistical algorithms to identify patterns, relationships, and trends within the data. By analyzing past data, predictive models can be built and applied to future scenarios or unseen data to predict outcomes or behaviors.
Supervised vs. Unsupervised Learning
In predictive modeling, there are two main types of learning: supervised and unsupervised. Supervised learning involves training a model using a labeled dataset, where the desired outcome or target variable is known. This type of learning is used for tasks such as classification and regression.
On the other hand, unsupervised learning deals with unlabelled data, where the model aims to find patterns or groupings in the data without prior knowledge of the outcomes. This type of learning is used for tasks such as clustering and anomaly detection.
Regression, Classification, and Clustering Algorithms
Regression, classification, and clustering are common tasks in predictive modeling and machine learning.
- Regression: Regression algorithms are used for predicting numerical or continuous values. They establish a relationship between the input variables and the target variable, allowing the model to make predictions.
- Classification: Classification algorithms are used when the target variable is categorical or discrete. They assign instances to predefined classes or categories based on the input variables.
- Clustering: Clustering algorithms are used to group similar instances together based on their characteristics or attributes. They help identify patterns or similarities in the data.
Overview of Popular Machine Learning Algorithms
In machine learning, there are various algorithms that are commonly used for different tasks.
- Linear Regression: Used for predicting a continuous target variable, given a set of input variables.
- Logistic Regression: Used for binary classification tasks, where the target variable has two possible outcomes.
- Decision Tree: Creates a tree-like model of decisions and their possible consequences.
- Random Forest: An ensemble of decision trees that improves the accuracy and robustness of predictions.
- k-means Clustering: Divides a dataset into k clusters based on similarity.
These are just a few examples of the numerous algorithms available in predictive modeling and machine learning.
Data Analytics Tools and Technologies
As a beginner in data analytics, it is important to familiarize yourself with the various tools and technologies that are commonly used in the field. These tools and technologies can greatly assist you in analyzing and interpreting data, allowing you to make informed decisions based on the insights gained.
Popular Tools and Software for Data Analytics
There are several popular tools and software available for data analytics, each with its own unique features and functionalities. Some of the most widely used tools include:
- Microsoft Excel: Excel is a basic tool that is commonly used for data analysis. It allows users to perform various calculations, create charts and graphs, and perform basic statistical analyses.
- Tableau: Tableau is a powerful data visualization tool that allows users to create interactive dashboards and reports. It enables users to easily explore and understand data through its user-friendly interface.
- Power BI: Power BI is another popular data visualization tool that is commonly used in data analytics. It offers a wide range of visualizations and allows users to create interactive reports and dashboards.
- Google Analytics: Google Analytics is an essential tool for analyzing website traffic and user behavior. It provides valuable insights into website performance and helps businesses optimize their online presence.
Overview of Programming Languages: Python, R, SQL
Programming languages play a vital role in data analytics, as they allow users to manipulate and analyze data. The following programming languages are commonly used in data analytics:
- Python: Python is a versatile language that is widely used in data analytics. It offers a robust set of libraries and packages, such as Pandas and NumPy, that simplify data manipulation and analysis.
- R: R is another popular programming language that is extensively used for statistical analysis and data visualization. It provides a wide range of packages specifically designed for data analytics.
- SQL: SQL (Structured Query Language) is a language commonly used for managing and manipulating databases. It allows users to retrieve, update, and analyze data stored in relational databases.
Big Data Analytics: Hadoop, Apache Spark, etc.
In today's data-driven world, big data analytics has gained immense importance. Big data refers to the large and complex datasets that cannot be easily managed and analyzed using traditional methods. To tackle big data challenges, various technologies and tools have been developed, including:
- Hadoop: Hadoop is an open-source framework that allows for the distributed processing of large datasets across clusters of computers. It provides a scalable and fault-tolerant solution for big data analytics.
- Apache Spark: Apache Spark is another popular big data analytics framework that offers fast and efficient data processing. It provides a unified analytics engine for big data processing, machine learning, and real-time streaming.
- Other Tools: Apart from Hadoop and Apache Spark, there are several other tools and technologies available for big data analytics, including Apache Cassandra, MongoDB, and Splunk.
Cloud-Based Analytics Platforms
Cloud-based analytics platforms have gained popularity in recent years due to their scalability, flexibility, and cost-effectiveness. These platforms allow users to store, manage, and analyze data in the cloud, eliminating the need for on-premise infrastructure. Some of the popular cloud-based analytics platforms include:
- Amazon Web Services (AWS): AWS offers a wide range of services for data analytics, such as Amazon Redshift for data warehousing and Amazon QuickSight for data visualization.
- Microsoft Azure: Microsoft Azure provides a comprehensive suite of tools and services for data analytics, including Azure Synapse Analytics and Power BI.
- Google Cloud Platform (GCP): GCP offers various services for data analytics, such as BigQuery for data warehousing and Data Studio for data visualization.
By familiarizing yourself with these data analytics tools and technologies, you will be well-equipped to embark on your data analytics journey as a beginner. Remember to choose the tools and technologies that best align with your specific needs and goals.
Ethical Considerations in Data Analytics
Data analytics has become an indispensable tool in various industries, providing valuable insights and driving informed decision-making. However, as the use of data analytics expands, it is crucial to address the ethical considerations associated with it. In this section, we will explore some of the key ethical considerations in data analytics.
Privacy and Data Protection
One of the primary concerns in data analytics is privacy and data protection. Organizations must ensure that the data they collect and analyze is kept confidential and used only for its intended purposes. This involves implementing appropriate security measures to safeguard data from unauthorized access or breaches.
Additionally, individuals must be informed about the types of data being collected and how it will be utilized. Consent must be obtained before collecting and using personal information, and individuals should have the right to access, modify, or delete their data.
Fairness and Bias in Data Analysis
Fairness and bias in data analysis are critical aspects to consider. Bias can be inadvertently introduced into the data through various stages of the analytics process, such as data collection, cleaning, and modeling. This can lead to unfair or discriminatory outcomes.
To address this, data analysts must be aware of potential biases and strive to eliminate or mitigate them. Using diverse and representative datasets, employing unbiased algorithms, and regularly reviewing and testing models for fairness can help ensure equitable outcomes.
Legal and Regulatory Compliance
Data analytics activities must comply with relevant laws and regulations to protect the rights and interests of individuals. Organizations must adhere to data protection regulations, such as the General Data Protection Regulation (GDPR), and ensure that they have appropriate legal grounds for collecting and processing data.
Compliance also involves respecting copyright and intellectual property laws when using external data sources or incorporating third-party analyses. Organizations need to establish clear policies and procedures to ensure compliance with applicable legal requirements.
Ensuring Ethical Use of Data
The ethical use of data encompasses a wide range of considerations, including transparency, accountability, and responsible data stewardship. Organizations should have clear policies and guidelines in place to govern data analytics practices and ensure ethical use.
This involves transparently communicating how data is used, providing individuals with control over their data, and regularly monitoring and auditing data analytics processes to detect and rectify any ethical violations. Organizations should also foster a culture of ethical awareness and ensure that employees are trained on ethical data handling.
Ethical considerations play a crucial role in data analytics. By addressing privacy, fairness, legal compliance, and ethical use of data, organizations can build trust, foster responsible data practices, and promote the long-term success of data analytics initiatives.
Challenges and Limitations of Data Analytics
Data analytics, while incredibly powerful and beneficial, is not without its challenges and limitations. As beginners in the field, it's important to be aware of these potential hurdles and understand how to overcome them.
Data Quality and Reliability
One of the most significant challenges in data analytics is ensuring the quality and reliability of the data being analyzed. Inaccurate or incomplete data can lead to flawed insights and incorrect conclusions. It's crucial to have robust data collection processes in place and to regularly validate and verify the data before using it for analytics.
Lack of Skilled Professionals
Another challenge is the scarcity of skilled professionals in the field of data analytics. As the demand for data analytics continues to grow, the need for professionals who possess the necessary skills and expertise also increases. Organizations often struggle to find qualified individuals who can effectively analyze data and derive meaningful insights. To overcome this challenge, aspiring data analysts should invest in acquiring the right skills and knowledge through specialized training programs and certifications.
Ethical and Legal Concerns
Data analytics involves handling and analyzing large amounts of personal and sensitive data. This raises ethical and legal concerns regarding privacy, data protection, and consent. It's essential for data analysts to understand and comply with relevant regulations such as the General Data Protection Regulation (GDPR) to ensure the ethical and secure handling of data. Organizational policies and procedures should also be in place to address these concerns and protect individuals' privacy rights.
Overcoming Limitations and Addressing Challenges
While challenges and limitations exist in data analytics, there are various strategies and approaches to overcome them. Organizations can invest in data quality improvement initiatives, such as data cleansing and data validation, to ensure high-quality data. Additionally, collaborating with specialized data analytics teams or partnering with external experts can help address the lack of skilled professionals. By adopting a proactive approach to tackle ethical and legal concerns, organizations can prioritize data protection and privacy and build trust with their customers and stakeholders.
Practical Examples and Case Studies in Data Analytics for Beginners
Looking for real-life examples and case studies in data analytics? You're in the right place. In this section, we will explore practical applications of data analytics and showcase successful projects that have leveraged its power.
Real-life examples of Data Analytics applications
- Marketing Analytics: Understanding customer behavior, analyzing campaign success rates, and optimizing marketing strategies based on data-driven insights.
- Financial Analytics: Predicting stock market trends, performing risk assessments, and detecting fraudulent activities using data analysis techniques.
- Healthcare Analytics: Analyzing patient data to identify patterns, improve patient care, and predict disease outbreaks.
- Social Media Analytics: Analyzing social media data to identify trends, improve engagement, and optimize content strategies.
Learning from practical use-cases to gain insights and knowledge
By studying practical examples and case studies in data analytics, beginners can gain valuable insights into how data analytics is applied in various industries and domains. These real-life use-cases provide a hands-on learning experience and help beginners understand the practical applications and benefits of data analytics.
Learning Data Analytics for a Beginner
Steps to start learning Data Analytics
Learning data analytics can seem overwhelming at first, but by following a systematic approach, you can build a strong foundation in this field. Here are some steps to get started:
- 1. Understand the basics: Begin by familiarizing yourself with the basic concepts and terminology in data analytics. This will help you grasp the fundamental principles and techniques used in this field.
- 2. Gain theoretical knowledge: Enroll in online courses or tutorials that provide comprehensive lessons on data analytics. These resources will teach you about various statistical methods, data visualization techniques, and predictive modeling concepts.
- 3. Practice with real-world examples: Hands-on experience is crucial in data analytics. Apply the techniques you've learned by working on practical projects and analyzing real datasets. This will help you develop the necessary skills and gain confidence in your abilities.
- 4. Stay up-to-date: Data analytics is a rapidly evolving field. Keep yourself updated with the latest trends, tools, and technologies by following industry blogs, attending webinars, and participating in online forums.
Essential skills and knowledge required
While learning data analytics, it is important to develop certain skills and acquire specific knowledge. Here are some essential skills and knowledge areas for a beginner:
- Statistical analysis: Understand basic statistical concepts such as probability, hypothesis testing, and regression analysis. This will form the basis for most analytical techniques.
- Data visualization: Learn how to present data visually using charts, graphs, and interactive dashboards. This skill is crucial for communicating insights effectively.
- Programming: Familiarize yourself with programming languages like Python or R, which are widely used in data analytics. This will enable you to manipulate data, build models, and automate tasks.
- Database management: Gain knowledge of SQL (Structured Query Language) to extract, manipulate, and analyze data stored in databases. This skill is essential for working with large datasets.
Online resources, courses, and certifications for beginners
There are many online resources available to help beginners learn data analytics. Here are some recommended platforms, courses, and certifications:
- Online platforms: Websites like Coursera, edX, and Udemy offer a wide range of data analytics courses and tutorials suitable for beginners.
- Google Analytics Academy: Google provides free online courses that teach data analytics using their analytics platform. This is a great resource for practical hands-on learning.
- DataCamp: DataCamp offers interactive courses that cover various aspects of data analytics, including data manipulation, visualization, and machine learning.
Building a career in Data Analytics
Data analytics offers exciting career opportunities for those interested in working with data and deriving meaningful insights. Here are some steps to begin building a career in this field:
- 1. Gain practical experience: While learning, take up internships or freelance projects to gain practical experience. This will not only strengthen your skills but also help you build a portfolio of work.
- 2. Networking: Connect with professionals in the field of data analytics through networking events, industry conferences, and online communities. Networking can open doors to job opportunities and mentorship.
- 3. Specialize in an area: Data analytics covers a wide range of domains. Identify your interests and specialize in a specific area such as business analytics, healthcare analytics, or marketing analytics.
- 4. Continuous learning: Data analytics is a dynamic field with new techniques and tools emerging constantly. Stay updated by continuously learning and acquiring new skills.
Conclusion
Throughout this guide, we have explored the world of data analytics for beginners, covering a wide range of topics and concepts. Let's recap some of the key points we've covered.
- We started with an introduction to data analytics, understanding its significance in today's data-driven world.
- We then delved into the basic concepts and terminology, ensuring a solid foundation for further learning.
- The importance and benefits of data analytics were highlighted, showcasing its role in making informed decisions and driving business growth.
- We explored various aspects of data analytics, including data collection, cleaning, preprocessing, exploratory data analysis, statistical analysis, hypothesis testing, data visualization, predictive modeling, and machine learning.
- We also discussed the ethical considerations that need to be taken into account when working with data.
- Challenges and limitations in the field of data analytics were addressed, emphasizing the need for continuous learning and adaptation.
- Practical examples and case studies were presented, providing real-world applications of data analytics for beginners to grasp.
- We provided guidance for beginners on how to learn data analytics and recommended tools and technologies to aid in their learning journey.
Now that you have a solid understanding of data analytics, it's time to dive into this exciting field. Don't be intimidated by the complexities that lie ahead – every data analyst started as a beginner.
Embrace the challenges and the opportunities that data analytics presents. This field is constantly evolving, which means there are endless possibilities for growth and development.
By learning data analytics, you will acquire a powerful skill set that can be applied to various industries and sectors. Whether you're interested in finance, healthcare, marketing, or any other field, data analytics will play a crucial role in shaping the future.
So, what are you waiting for? Start your journey in data analytics today and unlock a world of insights, discoveries, and endless career possibilities.
Frequently Asked Questions
The data analytics process typically involves defining objectives, data collection, data cleaning and preprocessing, exploratory data analysis, modeling and analysis, interpretation of results, and communicating findings.
Big data analytics enables businesses to analyze large volumes of diverse data (structured and unstructured) to uncover hidden patterns, trends, and correlations that traditional analytics might miss, leading to deeper insights and opportunities.
Common tools include programming languages like Python and R, data visualization tools like Tableau and Power BI, statistical software like SPSS and SAS, and database management systems like SQL Server and MySQL.
Data analytics helps marketers analyze customer behavior, segment audiences, measure campaign effectiveness, optimize marketing spend, and personalize messaging to improve engagement and ROI.
Data visualization transforms complex data into visual representations (charts, graphs, dashboards) that are easier to understand and interpret, facilitating insights and communication of findings.